What is deep learning?
A brief guide to deep learning – the phenomenon behind some of today's most advanced AI
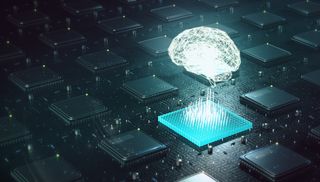
Machine learning has many broad methods, of which deep learning is perhaps the most well-known. A type of artificial intelligence (AI) technology, the ‘deep’ in deep learning refers to the layered and hierarchical nature of the algorithms it uses to train AI.
The real-world applications of deep learning are broad, but can most commonly be found in use cases such as voice recognition in smart home assistants, and natural language processing models used by AI-driven chatbots.
The ultimate ambition of deep learning is to teach an AI to make its own decisions based on the data it is fed. In the example of a smart assistant, to hard-code all the different types of questions a user could ask a home assistant would be nigh-on impossible and incredibly laborious. The more efficient, and perhaps more time-consuming in the short-term, method of building such an AI would be to train it using deep learning to recognise what it's being asked and how to appropriately respond.
Deep learning models are trained using data – tons of data. The more data that can be fed into a system, the more accurate the decisions it can make. Many deep learning models take inspiration from human biology, using neural networks that arrange analytical nodes in interconnecting pathways, allowing them to replicate the multi-layered (deep) connections found in the human brain.
Is deep learning supervised or unsupervised?
The special draw of deep learning that’s attracting so many to study the field is the potential for both supervised learning and unsupervised learning, although it's the latter that has opened up so many avenues for research.
Supervised deep learning definition
Referring to a training technique using labelled data, supervised learning is one approach researchers often take when training deep learning models. Ahead of training, data is prepped by researchers and labels are applied to both the input data and the output data. This is so the model can directly analyse the relationship between both sets. After the model has a definition of the relationship and once it understands why the relationship exists, it can start to more accurately analyse other bodies of data.
Such training is referred to as ‘supervised’ owing to the required presence of a human, whose main role is labelling the data so that the model can tell what it's processing. The purposes of this training are often seen in object detection applications and it's considered to be the most popular choice for model training in machine learning.
Get the ITPro. daily newsletter
Receive our latest news, industry updates, featured resources and more. Sign up today to receive our FREE report on AI cyber crime & security - newly updated for 2024.
Deep learning training can also be done in a semi-supervised manner which requires the labelling of just a small proportion of the overall training data set. A much greater amount of unlabelled data is then used simultaneously to analyze a problem.
Unsupervised deep learning definition
Unsupervised learning refers to the process of using entirely unlabelled data, and feeding it into the deep learning model with a view to training said model to recognise patterns in completely new data. The lack of human intervention creates potential for unforseen determinations to be made by the model, something that forms the basis of a huge body of research today.
There are three main tasks when it comes to unsupervised learning. These are:
- Clustering: Involves looking at unlabelled data and grouping similar data points into groups. This task is often used for training models to compress digital images, for example
- Association: Somewhat similar to clustering, the association approach is used to understand why there is a relationship between data points and how the data points connect rather than just knowing that there is a relationship there
- Dimensionality reduction: Often performed during the pre-processing of data, where researchers attempt to reduce the noise in the training data. This refers to ‘simplifying’ a given data set that has too many features, or dimensions, to accurately analyse. It involves reducing the number of data inputs so that a relationship between input and output data can be established
Examples of deep learning today
Neural networks commonly form the foundation of many advanced machine learning systems in the world today. The self-driving car, for example, has been made possible via deep learning, and the concepts of deep learning are also finding many applications in the defence and aerospace industries.
Deep learning has its limitations, however, despite its huge potential. Tasks that are more human-like, for example, hold back deep learning capabilities. Deep learning thrives more in the areas of pattern recognition, excelling in understanding the complicated but constant rules of the programming language Go. Even in pattern recognition, a huge amount of data is still required to train deep learning models.
Design a hybrid cloud platform that takes advantage of gen AI
Tasks of pattern recognition come to the fore most obviously in conversational AI systems, where deep learning constitutes a support network. Multimodal inputs are processed alongside multimodal outputs - think voice recognition capabilities and synthesized voices. Huge companies like Starbucks and Apple are already utilizing these sorts of capabilities, with customers now increasingly presented with opportunities to place orders with voice commands. Other applications include giving consumers the ability to log in to their phones just by looking at the screen.
At the current stage of development, it does not appear possible for deep learning to perform the same elaborate, adaptive thought processes as humans, however, the technology continues to evolve at quite a rate.
The future of deep learning
Deep learning may not result in killer robots anytime soon, but that isn't to say it won't fundamentally alter aspects of society in other ways.
Google Brain represented how its deep learning AI system was thinking for itself through an experiment involving cats. Though the research group did not specify any experimental parameters for the identification of cats, Google Brain was able to identify images of millions of cats.
While this may on the surface seem a banal use case, it's not hard to appreciate how this development could be put to a more practical use.
In medicine, deep learning has been found to be on par with human expertise when it comes to interpreting medical images. The study, carried out by the University of Birmingham, may pave the way for AI to play a greater role in the medical field going forwards, easing the strain on resources and allowing doctors to spend more time with patients.
Perhaps the most exciting area where deep learning is being touted as a possible springboard to discovery is in the cosmos. Researchers from ETH Zurich university released a paper in which they employed neural networks to study dark matter. When compared to the Hubble telescope, deep learning was found to deliver 30% more accurate values when breaking down the composites of the universe, apportioning baryonic matter, dark matter and dark energy. The researchers concluded by claiming that deep learning is a promising prospect for cosmological data analysis in the future.
What is certain, is that with funding being poured into AI - the Pentagon allocated nearly $1 billion to AI in 2020 - and specifically deep learning research studies, the technologies' influence will only grow.
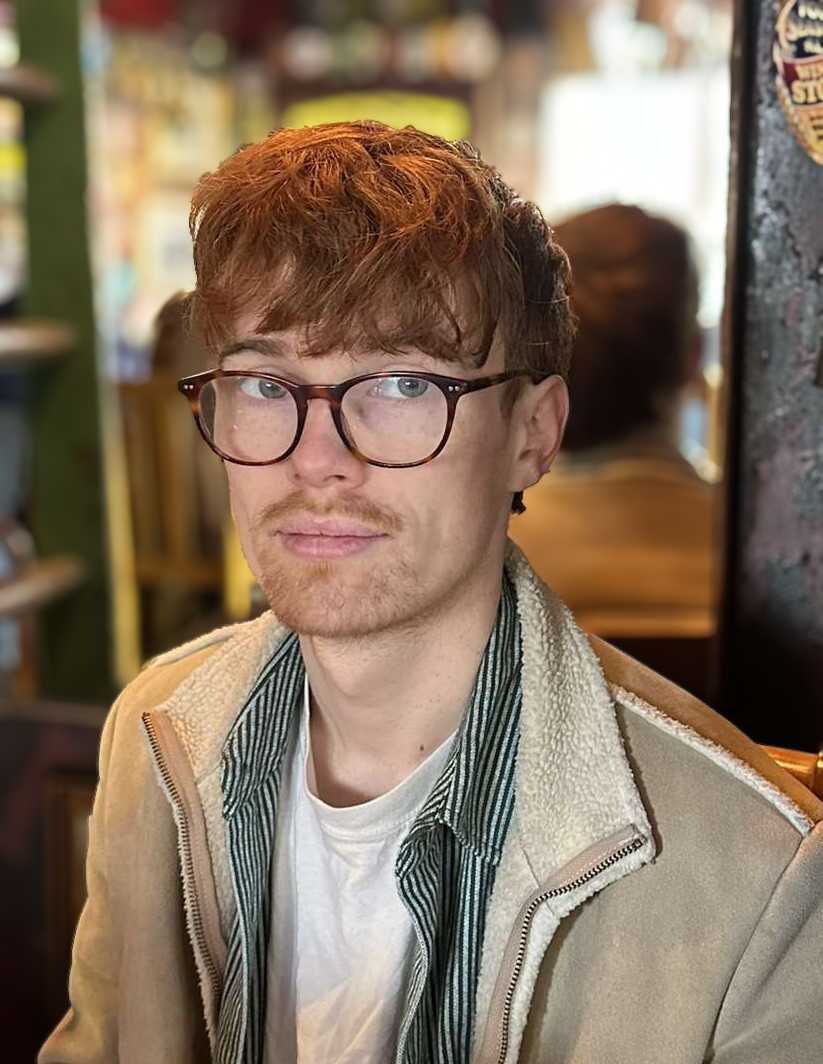
George Fitzmaurice is a staff writer at ITPro, ChannelPro, and CloudPro, with a particular interest in AI regulation, data legislation, and market development. After graduating from the University of Oxford with a degree in English Language and Literature, he undertook an internship at the New Statesman before starting at ITPro. Outside of the office, George is both an aspiring musician and an avid reader.